Bloomberg Quant (BBQ) Seminar Series – June 2024
Keynote Speakers:
• Mohammad Fesanghary | Quant Researcher, Bloomberg
• Achintya Gopal | Machine Learning Quant Researcher, Bloomberg
• Agathe Sadeghi | PhD Candidate, Stevens Institute of Technology
Causal Discovery in Financial Markets: What is it?
To gain a deeper understanding of financial markets, it is essential to grasp not only statistical but also causal dependencies. In this talk, we introduce the (basic) concepts of causal discovery which is how we can learn causal dependencies directly from data without randomized control trials (RCT). Even though we do not need to run an RCT, theory shows us that we do still need to make assumptions about the data for causal discovery to work. One of the most common is stationarity (e.g., Granger causality) which we know to be not true in finance.
We will also cover a new algorithm we developed called CD-NOTS (Constraint-based Causal Discovery from Nonstationary Time-Series) which is able to handle non-stationarity, non-linearity, non-Gaussianity, and lagged and contemporaneous effects, all of which are typical in financial data. Finally, we show the results of CD-NOTS on real data and show that our algorithm is a more effective alternative to other causal discovery algorithms.
6:30 PM
Lightning Talks
-Revant Nayar | FMI Technologies
Quant Lessons from 20th Century Physics
-Lily Gu | Bloomberg
Automated Market Makers
-Natascha Hey | Ecole Polytechnique
Trading with Concave Price Impact
-Philip Lasser | The Juilliard School
Beethoven’s 9th Symphony: From Motive to Structure
Speakers
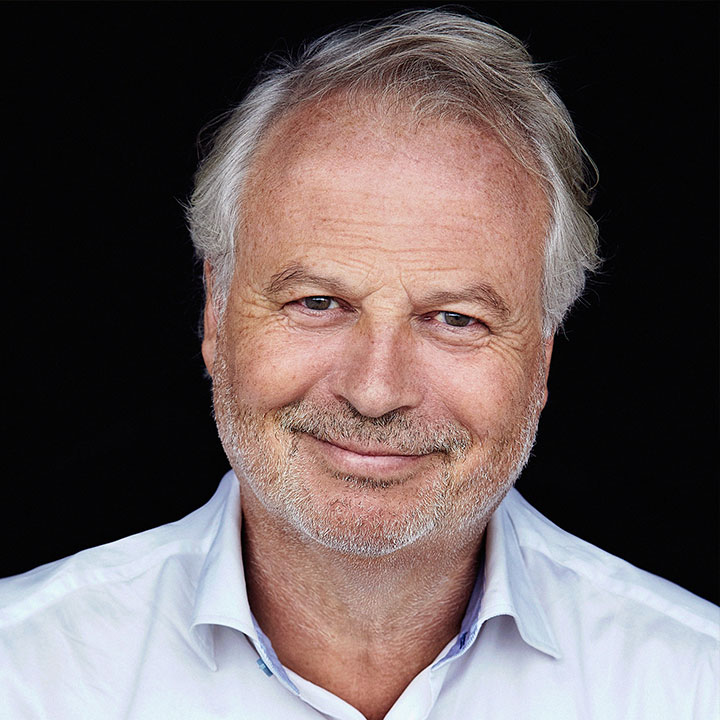
Bruno Dupire
Global Head of Quantitative Research, CTO Office
Bloomberg
Bruno Dupire is the Global Head of Quantitative Research, CTO Office at Bloomberg, which he joined in 2004. Prior to this assignment in New York, he has headed the Derivatives Research teams at Société Générale, Paribas Capital Markets and Nikko Financial Products where he was a Managing Director. He is best known for having pioneered the widely used Local Volatility model (simplest extension of the Black-Scholes-Merton model to fit all option prices) in 1993 and the Functional Itô Calculus (framework for path dependency) in 2009. He is a Fellow and Adjunct Professor at NYU and he is in the Risk magazine “Hall of Fame”. He is the recipient of the 2006 “Cutting edge research” award of Wilmott Magazine and of the Risk Magazine “Lifetime Achievement” award for 2008. He runs and organizes the Bloomberg Quant (BBQ) seminar, the largest monthly event of this kind.
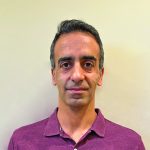
Mohammad Fesanghary
Quant Researcher
Bloomberg
Mohammad Fesanghary is a quantitative researcher at Bloomberg with over 10 years of experience in data science, operations research, and machine learning. He holds a Ph.D. in engineering from LSU, where he made significant contributions to metaheuristic optimization algorithms. His research, cited by thousands of researchers and practitioners, has found wide applications across various industries. Currently, Dr. Fesanghary focuses on causal discovery, causal inference, and time series analysis, particularly within the context of finance.
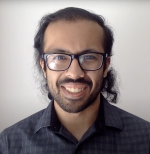
Achintya Gopal
Machine Learning Quant Researcher
Bloomberg
Achintya Gopal is a Machine Learning Quant Researcher in the Quantitative Research group in Bloomberg’s Office of the CTO, where he works on applying machine learning within the financial domain. Prior to this, he worked on estimating carbon emissions using machine learning, developing new models in normalizing flows, and exploring new methods to evaluate statistical models with model uncertainty. More recently, he has worked on a variety of projects related to volatility modeling using neural networks, causal inference for investing, generative models in differential privacy, active learning for NLP, the interpretability of large language models, and generative modeling for factor analysis.
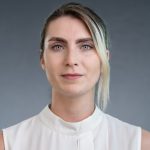
Agathe Sadeghi
PhD Candidate
Stevens Institute of Technology
Agathe Sadeghi is a PhD candidate in Financial Engineering at Stevens Institute of Technology, supervised by Dr. Zachary Feinstein. Her research focuses on network theory, centrality measures, and contagion risk, with applications in Value at Risk and Expected Shortfall estimation and portfolio management. She also explores causal network structures and inference using Machine Learning to identify potential causal drivers of different asset classes. Additionally, Agathe is an instructor at Stevens, where she teaches the financial database course to graduate students.