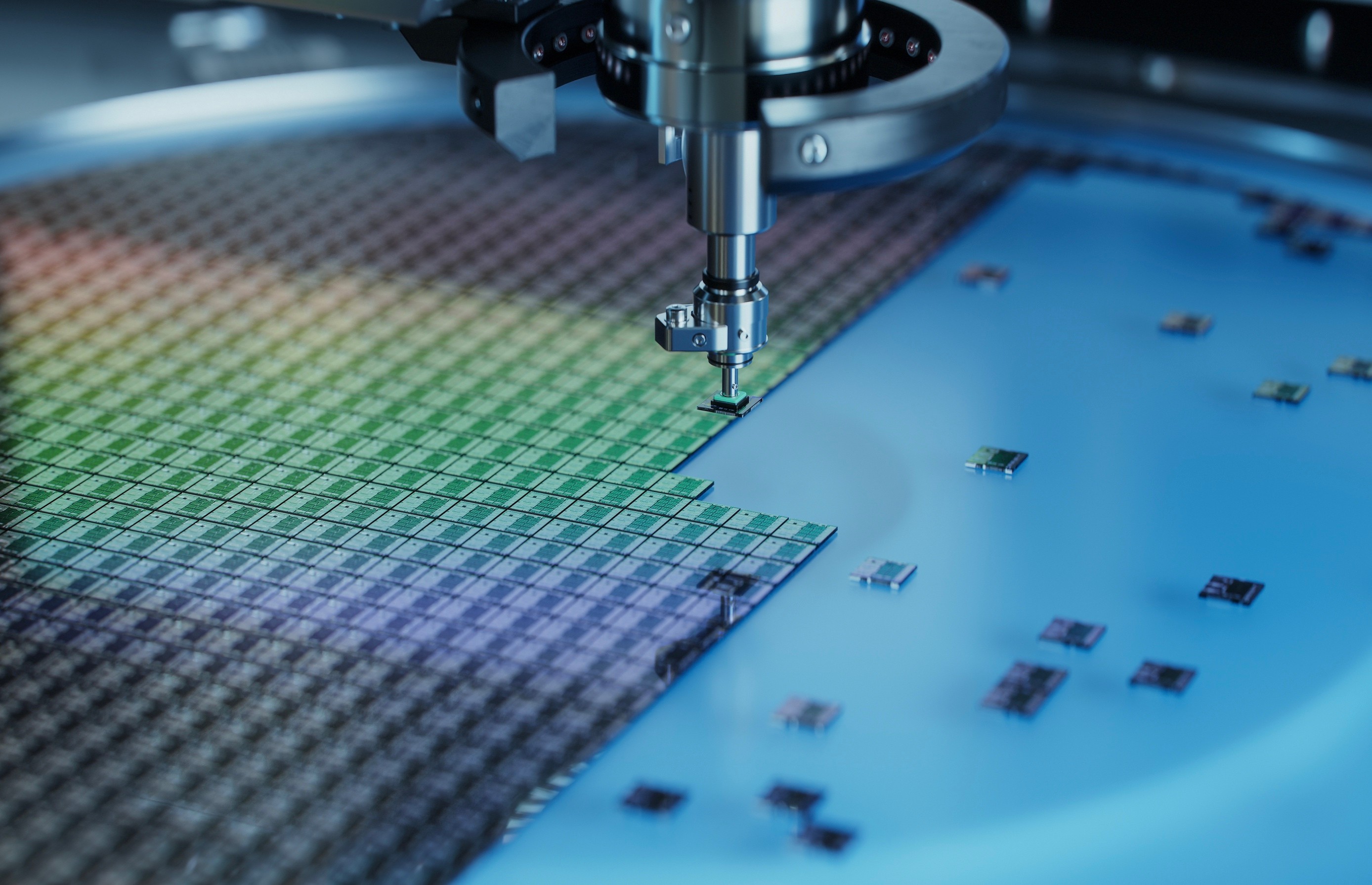
Bloomberg News
This article was written by Seth Fiegerman and Nate Lanxon, with contributions by Dina Bass, Jackie Davalos, Shirin Ghaffary, and Rachel Metz . It appeared first on the Bloomberg Terminal.
Every advance in artificial intelligence comes with a confusing plethora of arcane terminology. Here’s a guide to distinguish your AGIs from your GPTs.
The arrival in late 2022 of the ChatGPT chatbot, with its remarkably sophisticated — if occasionally erroneous — answers to a vast array of queries, was a milestone in artificial intelligence that took decades to reach. Scientists were experimenting with “computer vision” and giving machines the ability to “read” as far back as the 1960s, and chatbots began life when the Beatles were still making music.
Now tech companies are racing to develop ever more sophisticated AI products that can talk back to users, solve complex math problems, produce short films and perhaps one day outperform a human in a wide variety of tasks. Whether you’re worried about being replaced by a machine, or just intrigued by the possibilities, here’s the terminology you need to navigate an AI-driven world.
- AGI: AI companies are obsessed with the idea of artificial general intelligence, or AGI. But none of them can quite agree on how to define it. The term typically refers to hypothetical AI systems that are capable of completing a wide range of complex tasks with little human involvement. ChatGPT developer OpenAI goes a step beyond this and defines AGI as “highly autonomous systems that outperform humans at most economically valuable work.” But it’s not clear what counts as a “highly autonomous system,” or for that matter, “economically valuable work.” Some in the AI industry think we’ll reach AGI within the next decade; others believe it’s much further out, if it ever happens.
- agents: If the first year or so of the generative AI frenzy was defined by chatbots, the next phase may be defined by agents. That, at least, is the bet that many tech companies are making. Chatbots like ChatGPT may be able to spit out a quick recipe or a list of restaurants, but the hope is that AI agents will be able to order groceries for you or make a restaurant reservation on your behalf. While this may be appealing for personal and professional uses, it also raises the stakes for when AI makes an error.
- algorithm: An algorithm is a step-by-step process used to solve a problem. Take an input, apply some logic and you get an output. Humans have been using algorithms to solve problems for centuries. Some financial analysts spend their careers building algorithms that are able to predict future events and help them to make money. Our world runs on these “traditional” algorithms, but recently there has been a shift toward “machine learning,” which builds on these ideas.
- alignment: To prevent AI from running amok, some in the industry are focused on solving the problem of alignment — or making sure the technology is built to act in accordance with core human values. One problem, however, is that not everyone agrees on what those values are, or what AI systems should and should not be allowed to do.
- artificial intelligence: The broad term gets tossed around so much that it loses some of its meaning. At a high level, however, artificial intelligence refers to technology that models human intelligence and can perform a range of tasks that might otherwise have required people to handle. Computer scientist John McCarthy coined the term AI in the 1950s, but it didn’t take off in earnest until this century, when technology giants such as Google, Facebook owner Meta Platforms Inc. and Microsoft Corp. combined vast computing power with deep pools of user data. While AI can show humanlike abilities in data processing or conversation, the machines don’t yet “understand” what they’re doing or saying. They’re still relying essentially on algorithms.
- benchmarks: Given the increasingly crowded market for AI services, tech companies typically cite a range of benchmarks to show how their software outranks the competition. But there is still no independent, standardized test that AI companies use to compare how their software stacks up. Some in the industry are trying to fix this problem. For now, companies typically design their own benchmarks to show how well their services respond to questions about algebra, reading comprehension and coding.
- chatbots: Chatbots predate the rise of generative AI, as anyone trying to connect with customer service online knows. But a new era of AI chatbots is able to hold more dynamic exchanges with people on topics ranging from historical trivia to new food recipes. And as companies such as OpenAI and Google invest in more sophisticated models, chatbots are likely to become even more useful and conversational, perhaps approaching the tech industry’s longtime goal of an all-purposes virtual personal assistant.
- Claude: Claude is one of the few services to truly rival the performance of OpenAI’s most advanced technology. The chatbot was developed by Anthropic, a startup founded by a group of former OpenAI employees with a focus on prioritizing the safe development of artificial intelligence. Like ChatGPT, Claude can respond quickly to a range of queries from users. But unlike OpenAI, Anthropic has so far avoided certain use cases such as image generation. The startup says it’s focused on building products primarily for business customers.
- computer vision: A field of AI that allows computers to scan visual information such as images and video, identifying and classifying objects and people. The systems can react to what they see and take or recommend a particular action. The technology is being used to track wildlife for conservation and guide autonomous vehicles. There’s been concern about its use in military operations and policing, where it’s been shown to exhibit racial bias and to lack the precision needed to reliably identify a particular person.
- emergent behaviors: As large language models reach a certain scale, they sometimes start to display abilities that appear to have emerged from nowhere, in the sense that they were neither intended nor expected by their trainers. Some examples include generating executable computer code, telling strange stories and identifying movies from a string of emojis as clues.
- fine-tuning: Think of it as a fancy term for customization. With fine-tuning, a user takes an existing AI model and trains it on additional information about a particular task or subject area. This can help the model perform the way the user wants. For example, a company that sells exercise equipment might choose to fine-tune an AI model to better respond to queries about proper maintenance for an exercise bike.
- frontier models: Frontier models refer to the most advanced AI models on the market. At the moment, the companies behind these models include OpenAI, Anthropic, Google and Meta — all of which are part of a group called the Frontier Model Forum focused on working with academics and policymakers to promote responsible development of cutting-edge AI systems. The cost of developing these cutting-edge models is expected to grow significantly, making it harder for startups to compete with bigger tech companies.
- Gemini: An early frontrunner in the AI race, Google is now fighting to keep pace with OpenAI. The centerpiece of Google’s effort is Gemini, the name given to its flagship chatbot and its family of AI models. The most advanced version of Gemini, called Ultra, is pitched as being able to handle complex coding tasks and mathematical reasoning — similar to the most state-of-the-art version of OpenAI’s technology. Google has built multimodal capabilities into Gemini, allowing the AI model to, say, respond to an image of a meal with a recipe for how to make it.
- generative AI: This refers to the production of works — pictures, essays, songs, sea shanties — from simple questions or commands. It encompasses the likes of OpenAI’s DALL-E, which can create elaborate and detailed images in seconds, and Suno, which generates music from text descriptions. Generative AI creates a new work after being trained on vast quantities of preexisting material. It’s led to some lawsuits from copyright holders who complain that their own work has been ripped off.
- GPTA: generative pretrained transformer is a type of large language model. “Transformer” refers to a system that can take strings of inputs and process them together rather than in isolation, so that context and word order can be captured. This is important in language translation. For instance: “Her dog, Poppy, ate in the kitchen” could be translated into the French equivalent of “Poppy ate her dog in the kitchen” without appropriate attention being paid to order, syntax and meaning.
- Grok: At first blush, it’s easy to discount Grok as an unserious effort. The chatbot, built by Elon Musk’s AI startup xAI and available to subscribers on his X microblogging platform, has made headlines for its irreverent written responses and for spitting out incendiary images with few clear guardrails. But xAI has raised billions, attracted a talented team and has access to a vast trove of data from X users that it can use to build its AI products. As a result, Grok has emerged as a genuine competitor in a remarkably short period of time.
- hallucination: When an AI service like ChatGPT makes something up that sounds convincing but is entirely fabricated, it’s called a hallucination. It’s the result of a system not having the correct answer to a question but nonetheless still knowing what a good answer would sound like and presenting it as fact. There’s concern that AI’s inability to say “I don’t know” when asked something will lead to costly mistakes, dangerous misunderstandings and a proliferation of misinformation. Some AI companies say they’ve been able to improve accuracy with more recent models, including by having chatbots take more time to reason before responding to prompts, but the problem of hallucinations persists.
- large language models: These are very large neural networks that are trained using massive amounts of text and data, including e-books, news articles and Wikipedia pages. With billions of parameters to learn from, LLMs are the backbone of natural language processing that can recognize, summarize, translate, predict and generate text.
- Llama: Meta invested heavily to build Llama, a group of cutting-edge AI models that it’s making freely available for other developers to access and build upon. With this approach, Meta hopes Llama will be the foundation not just for its own chatbot, Meta AI, but also for a long list of products from other companies. That could put Meta — and Llama — at the center of the AI ecosystem.
- machine learning: This is the process of gradually improving algorithms — sets of instructions to achieve a specific outcome — by exposing them to large amounts of data. By reviewing lots of “inputs” and “outputs,” a computer can “learn” without necessarily having to be trained on the specifics of the job at hand. Take the iPhone photo app. Initially, it doesn’t know what you look like. But once you start tagging yourself as the face in photos taken over many years and in a variety of environments, the machine acquires the ability to recognize it.
- model collapse: Researchers have found that when AI models are trained on data that includes AI-generated content — something that’s increasingly likely given how much of that content now circulates online — they eventually end up with deteriorated performance. Some AI-watchers have raised concerns that these models may even “collapse” if they are trained on too much content generated by AI. A 2023 study of model collapse showed that AI images of humans became increasingly distorted after the model retrained on “even small amounts of their own creation.”
- multimodal: Increasingly, AI companies are focusing on “multimodal” systems that can process and respond to a range of inputs, including text, images and audio. For example, you might be able to speak to a chatbot and have it speak back, or show it an image of a math problem and ask for a solution. Not only does this boost the versatility of AI products, it also feels more like a real conversation with a digital assistant.
- natural language processing: This branch of AI helps computers to understand, process and generate speech and text the way a human would. NLP relies on machine-learning algorithms to extract data from written text, translate languages, recognize handwritten words, and discern meaning and context. It’s the underlying technology that powers virtual assistants like Siri or Alexa and allows them to not only understand requests but also respond in natural language. NLP can also gauge emotion in text, which is why if you tell Siri “I’m sad” it might suggest you call a friend. Other everyday applications include email spam filtering, web search, spell checking and text prediction.
- neural networks: This is a type of AI in which a computer is programmed to learn in very roughly the same way a human brain does: through trial and error. Success or failure influences future attempts and adaptations, just as a young brain learns to map neural pathways based on what the child’s been taught. The process can involve millions of attempts to achieve proficiency, which is one reason why AI platforms require vast amounts of computer processing power.
- open source: One of the key divides in the AI industry — and among those looking to regulate it — is whether to embrace open or closed models. While some use the term “open” loosely, it refers to the idea of open-source models, whose developers make their source code freely available for anyone to use or modify it. The definition comes from the nonprofit Open Source Initiative, which notes that truly open-source software must comply with specific terms for distribution and access.
- parameters: When an AI company releases a new model, one key figure it will often cite to differentiate the product is the number of parameters. The term refers to the total number of variables picked up by a model during the training process and serves as an indication of just how large a large language model really is. The numbers can be pretty staggering: For example, Meta’s Llama AI model comes in three sizes, with the largest having roughly 400 billion parameters.
- prompt: The experience of using today’s AI tools usually starts with a prompt — essentially any query or request from a user. Examples of prompts might include asking an AI chatbot to summarize a document, suggest home renovation tips or come up with a song lyric about falling in love with blueberry muffins.
- prompt engineering: The accuracy and usefulness of an AI platform’s responses depends to a large extent on the quality of the commands it is given. Prompt engineers can fine-tune natural-language instructions to produce consistent, high-quality outputs using minimum computer power.
- reasoning: In September 2024, OpenAI began rolling out a new model that can perform some humanlike reasoning tasks, such as responding to more complicated math and coding problems. In essence, the updated AI system takes more time to compute an answer before responding to the user, enabling it to better solve multistep problems. Google and Anthropic are also developing reasoning skills with their advanced AI models.
- small models: After years of one-upping each other to build larger models, some in the tech AI industry have embraced the idea that bigger isn’t always better. OpenAI, Google, Meta and others have all released small models — software that is more compact and nimble than their flagship large language models. While these options may not outperform larger alternatives, small models can be a more efficient and affordable option for customers.
- sentient AI: Most researchers agree that a sentient, conscious AI — one that’s able to perceive and reflect on the world around it — is years from becoming reality. While AI can display some humanlike abilities, the machines don’t yet “understand” what they’re doing or saying. They are just finding patterns in the vast amounts of information generated by human beings and generating formulas that dictate how they respond to prompts. And it may be hard to know when sentience has arrived, as there’s still no broad agreement on what consciousness is.
- synthetic data: In the push to find ever more data to develop the large language models that power AI chatbots, some tech companies are experimenting with synthetic data. Companies use their own AI systems to generate writing and other media that can then be used to train new models. The benefit of this approach is that it avoids some of the legal and ethical concerns around where training data is sourced from. But there may be a catch: Some worry it could lead to AI systems having deteriorated performance — the phenomenon known as model collapse.
- training data: AI companies scrape or license vast amounts of data in order to develop — or train — AI models that can spit out text, images, music and other media in response to queries from users. The companies tend to be tight-lipped on spelling out the specific training data they rely on, but for an AI chatbot, it might include articles, books, online comments and social media posts. Suno, an AI music generator, said its software was trained on “tens of millions of recordings,” including work that may be copyrighted.