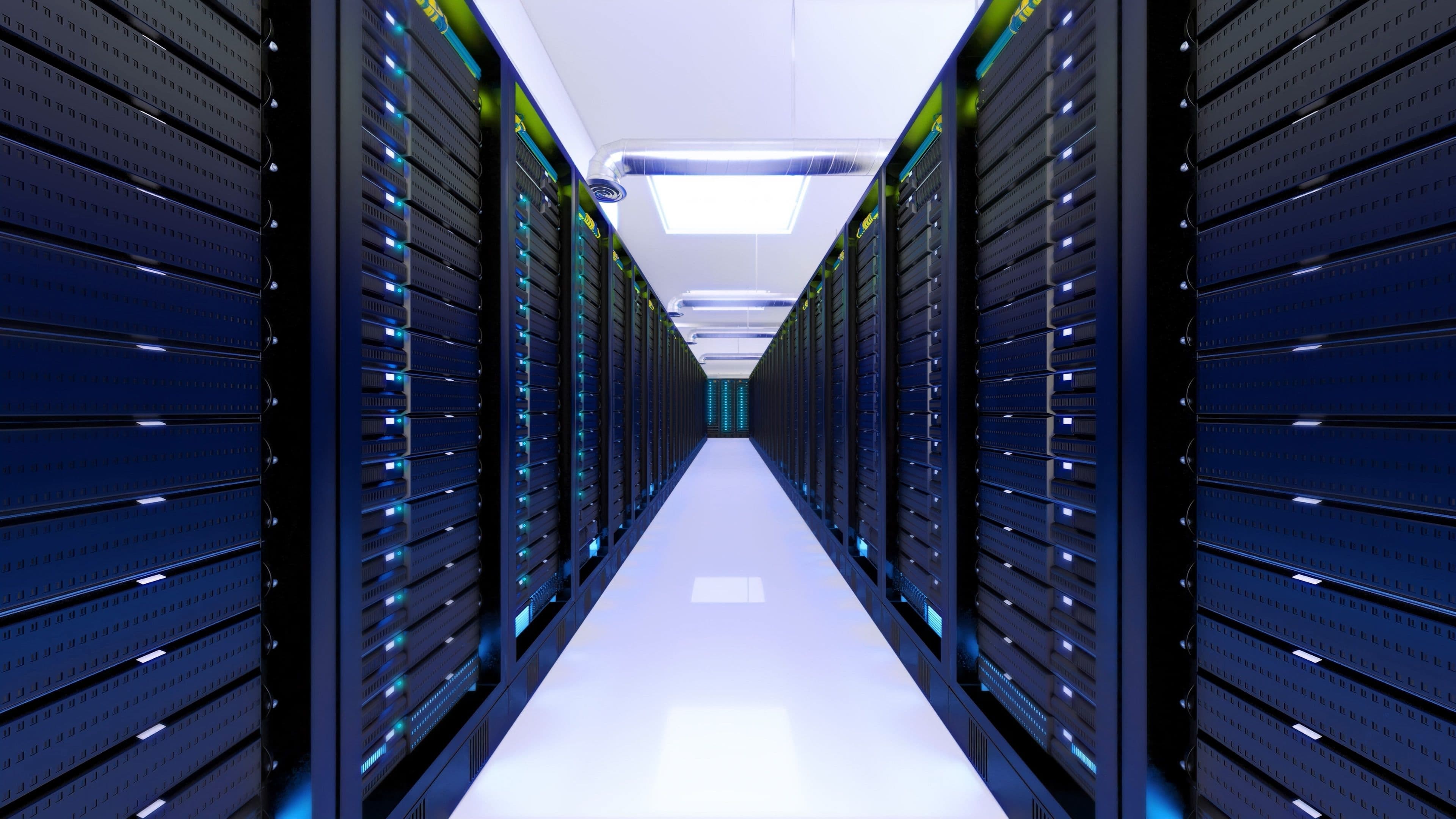
Bloomberg Intelligence
This analysis is by Bloomberg Intelligence Senior Industry Analyst Mandeep Singh. It appeared first on the Bloomberg Terminal.
The continuous scaling of foundational AI models will be a tailwind for both cloud hyperscalers and companies licensing their large language models for broader enterprise use. We expect capital spending on LLM training and inferencing to remain robust over the medium term, reaching around $1.13 trillion dollars through 2032. The high barriers to train a foundational model has already driven consolidation among LLM providers.
Training costs for LLMs
Training of LLMs remains the largest component of the Bloomberg Intelligence Gen AI forecast through 2032. With the five leading foundational-model providers — OpenAI, Google, Meta, Anthropic and Mistral — focused on scaling the next version of their LLMs using the latest GPUs, we expect training costs to expand in proportion with the growth in AI model parameters. Though small language models such as GPT-4o mini, Gemini and Llama versions have been released for on-device and edge deployment, the release of OpenAI’s chain-of-thought reasoning o1 model suggests that investments in building out frontier models are likely to continue in the near to medium term.
Training costs are expected to reach $646 billion, while inferencing could be $487 billion, with growth weighted to the back end, based on our BI market sizing model.
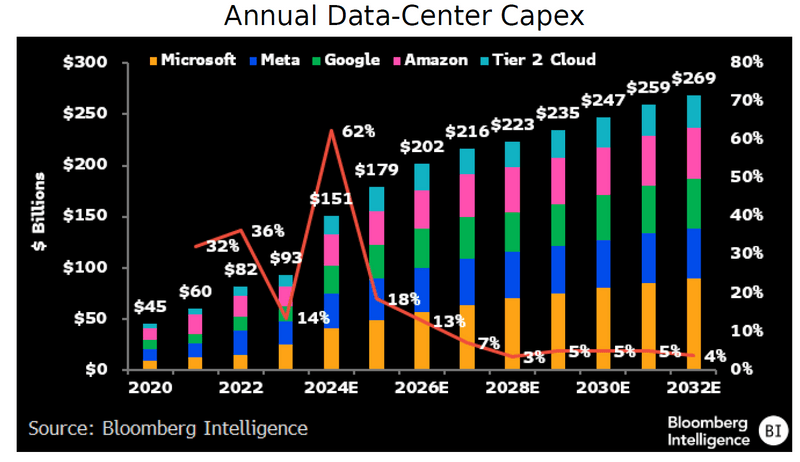
Capital spending poised to remain high
Data-center capex is likely to stay robust for hyperscale cloud providers like Microsoft, Amazon.com, Google and Oracle, whose AI workloads have contributed multibillion dollars in revenue to their cloud-infrastructure businesses. Microsoft’s Azure AI segment is already at a run-rate of $5 billion, a high-single-digit contribution to Azure segment sales of around $80 billion. Similarly, Amazon and Google have called out multibillion-dollar sales addition from AI infrastructure.
Though investments in data-center expansion are likely behind the more than 50% hyperscaler capex gains for 2024, we believe spending growth will taper gradually, given the faster architecture releases of GPUs and strong demand for both AI training and inferencing.
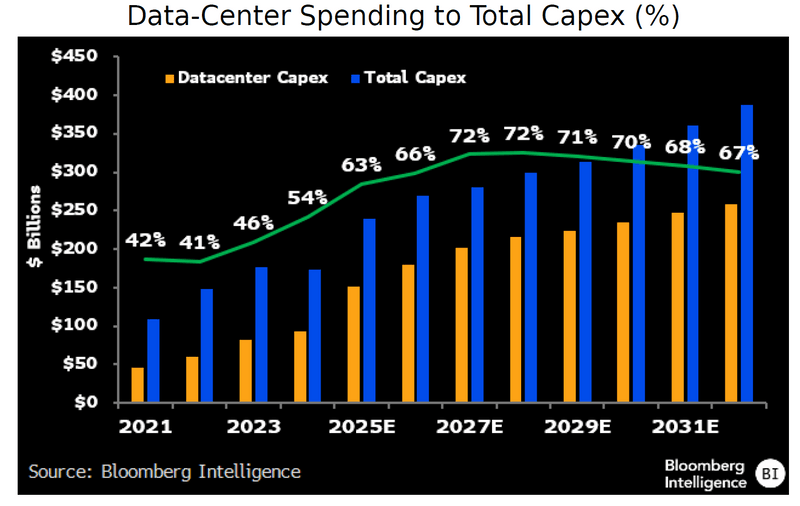
Bigger cluster sizes for training
While GPT-4 was trained using a cluster of 25,000-30,000 A100 GPU chips, costing about $300 million for one training run, the newer models will likely require a bigger-sized cluster proportional to the growth in model parameters. Xai is building a 100,000 H100 cluster, while Nvidia CEO Jensen Huang has painted a vision for connecting one million GPUs in an AI factory. OpenAI expects to spend more than $200 billion through 2030, based on reporting from The Information, the majority of which will be spent on training its LLM.
Meta is using its growing GPU compute clusters to power the training and deployment of its Llama LLM across its family of apps. Hyperscalers including Microsoft, Amazon, Google and Oracle have seen their cloud segments’ growth accelerate, driven by AI-infrastructure workloads.
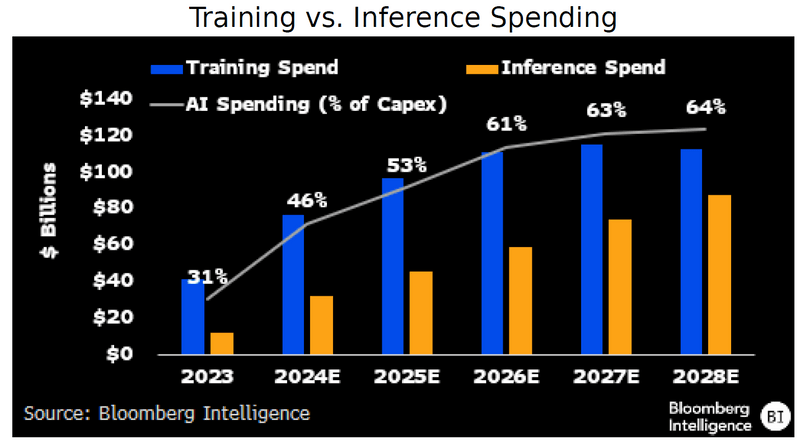
Model training concentration grows
Recent talent acquisitions by hyperscalers including Google-Character AI, Microsoft-Inflection and Amazon-Adept AI suggest continued consolidation among the foundation model companies. Given the continuous scaling of LLMs and elevated capex requirements for data centers needed to deploy generative AI, we expect very high barriers to entry for companies looking to train their own foundational models.
With the supply constraints around the latest GPUs, which have at least 2-3x performance and computation advantage over the prior generation, we believe hyperscalers are unlikely to pull back on data-center spending through 2026, barring a plateauing of LLM parameters or model performance.
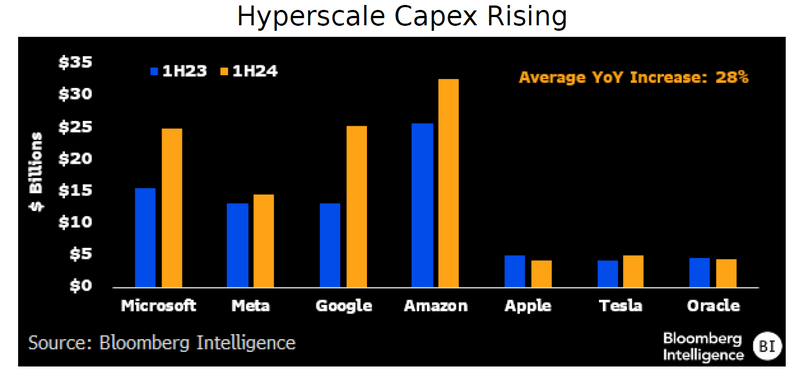
Apple vs. big tech peers
Apple continues to maintain the lowest capex intensity among big tech peers. That’s partly due to the fact that it isn’t a hyperscale cloud vendor that can leverage its distribution to support multiple LLMs. Capex intensity for Google and Meta could remain around 15-20% for the medium term, as these companies seek to scale the next version of their AI models. Though capex growth is likely to slow gradually over the next five years, a boost in cloud revenue could offset any headwinds to free cash flow.
Pure-play gen AI LLM vendors like OpenAI, Anthropic and Mistral are likely to rely on licensing sales while partnering with hyperscale cloud providers to offset pressure from the elevated costs of AI training.
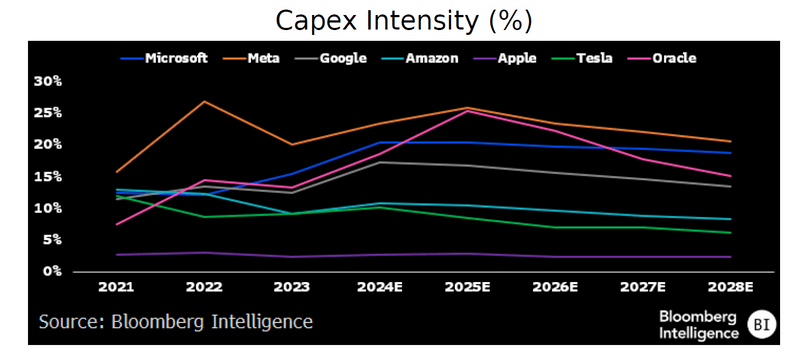
The data included in these materials are for illustrative purposes only. The BLOOMBERG TERMINAL service and Bloomberg data products (the “Services”) are owned and distributed by Bloomberg Finance L.P. (“BFLP”) except (i) in Argentina, Australia and certain jurisdictions in the Pacific Islands, Bermuda, China, India, Japan, Korea and New Zealand, where Bloomberg L.P. and its subsidiaries (“BLP”) distribute these products, and (ii) in Singapore and the jurisdictions serviced by Bloomberg’s Singapore office, where a subsidiary of BFLP distributes these products. BLP provides BFLP and its subsidiaries with global marketing and operational support and service. Certain features, functions, products and services are available only to sophisticated investors and only where permitted. BFLP, BLP and their affiliates do not guarantee the accuracy of prices or other information in the Services. Nothing in the Services shall constitute or be construed as an offering of financial instruments by BFLP, BLP or their affiliates, or as investment advice or recommendations by BFLP, BLP or their affiliates of an investment strategy or whether or not to “buy”, “sell” or “hold” an investment. Information available via the Services should not be considered as information sufficient upon which to base an investment decision. The following are trademarks and service marks of BFLP, a Delaware limited partnership, or its subsidiaries: BLOOMBERG, BLOOMBERG ANYWHERE, BLOOMBERG MARKETS, BLOOMBERG NEWS, BLOOMBERG PROFESSIONAL, BLOOMBERG TERMINAL and BLOOMBERG.COM. Absence of any trademark or service mark from this list does not waive Bloomberg’s intellectual property rights in that name, mark or logo. All rights reserved. © 2024 Bloomberg.